Airport Series: Chicago And Viral Reputation Management
In this installment of the airport series, we turn to Chicago O'Hare. ORD is still feeling repercussions after a viral incident from early 2017 rocked its customer base. This PR nightmare emphasizes an important role of text analytics as an agent of brand management.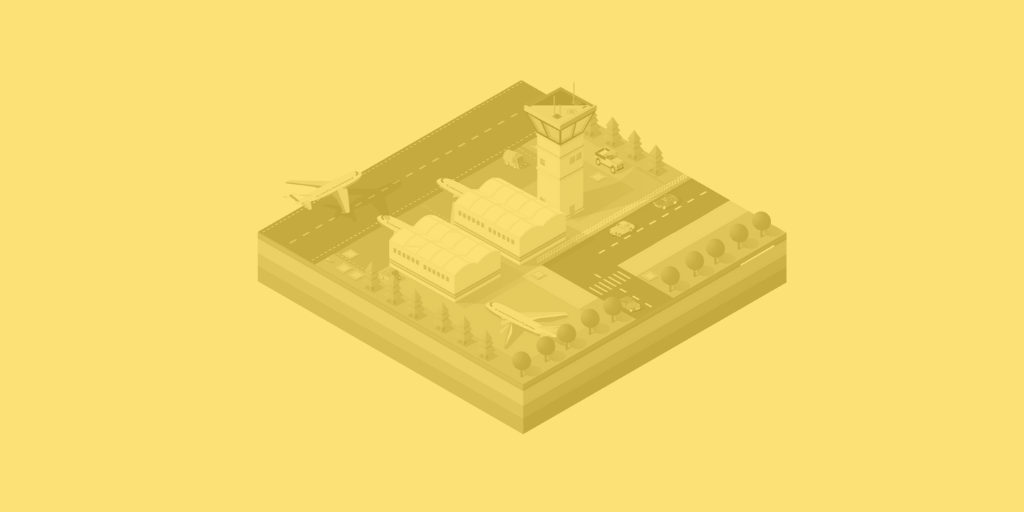
As we continue our ongoing analysis of airport reviews, we come to Chicago O’Hare International Airport. The story of Chicago’s airport is a study in how not to manage your reputation in the face of a viral public relations disaster.
Chicago O’Hare and viral reputation management
On April 9, a video of Dr. David Dao being violently dragged from a United Airlines flight went viral and made national news. Eventually, two municipal aviation officers were fired. United Airlines wasn’t fined, but they did take a major public relations hit. I’ve already written about public reaction from a high-level viewpoint. But when looked at more closely, O’Hare’s proximity to the incident is a study in viral reputation management.
“I think after watching videos of [people] getting dragged off planes at your airport…I pray you go BANKRUPT!!!” wrote one angry user.
Said another:
“I’m not flying through anywhere that condones its airport police staff assaulting a flyer…. I’ll avoid it completely. I understand you suspended one of these highly trained officers, my question is why haven’t you fired him!”
A full 20% of these comments urge potential customers to eschew O’Hare in favor its chief competitor, Midway. Further still, some social media users recommend routing layovers through an entirely different state. “Use Mitchell Airport in Milwaukee (MKE). No Beatings at Mitchell,” one user wrote, adding that people who wanted to “avoid assaults” should go there. What we see from our analysis is just how far from “engaged” and “contented” O’Hare’s guests are.
Responding with Analytics
By using a natural language processing solution to monitor their social media, Chicago O’Hare might’ve gotten ahead of the public vitriol with a proactive, rapid-response PR blitz. (Incidentally, there’s only one other complaint of equal volume on Chicago O’Hare’s Facebook reviews: WiFi. O’Hare only offers 30 minutes of free WiFi. As one tourist from Italy put it:
“Only 30 min free wifi! Not enough for USA concept of freedom.”
But instead, they were put on the back foot and forced into taking reactionary measures.
In fact, we can see the topic trending through April using Lexalytics’, an InMoment company, web dashboard, Semantria Storage & Visualization. Occurrences of Facebook ratings with 1 star within the data set jump from 11% to 58% in a matter of days. Meanwhile, reviews with a 5 star rating halve from 37% to less than 15% overnight. ORD’s negative social conversation dominates its Facebook feed for months later, with 1 star reviews maintaining a 2:1 ratio over 5 star reviews until August 2018.
Guilty by brand association
Why is this relevant? Even though Dr. Dao’s removal isn’t Chicago O’Hare’s fault, it still casts a pall over their brand. In the words of the legendary advertising firm Ogilvy, “a brand is guilty by association.” What’s more, the public is not a court, and its judgement is often unequivocal and uncompromising. Addressing this judgement head-on is often the only way to mitigate it.
Considering how the internet played the role of catalyst in this PR crisis, O’Hare might’ve used the opportunity to debut free WiFi in its facilities. They could’ve positioned it as a strategy to empower guest feedback at all times. Connections like this can open up a line of communication between the brand and the customer, potentially easing tensions. In this example, enabling free WiFi speaks to two public concerns at once.
“…O’Hare’s proximity to the incident is a study in viral reputation management.”
Chicago O’Hare needs text analytics
It turns out Chicago O’Hare didn’t take any dramatic steps to curtail public outcry. This could turn out to be a folly that comes back to haunt them. As it stands, airports play in a high-risk space where profit is lean at best. In fact, 70% of airports lose money. As regulations and other burdens pile up, airports like O’Hare need to depend less on aeronautical revenue and more on non-aeronautical revenue, such as retail developments, office developments, and lifestyle developments—like WiFi. However, non-aeronautical revenue is contingent upon engaged and contented customers. What we see from our analysis is just how far from “engaged” and “contented” O’Hare’s guests are.
A whopping 87% of O’Hare’s Facebook reviews from 2017 bear sentiment weight somewhere between negative and neutral. That’s a grim place for any brand. But it’s not a total loss; a simple application of tools like text analytics can solve reputation woes like O’Hare’s affordably and effectively. These are technological solutions that can’t be neglected. When it comes to an asset-intensive business like the modern airport, text analytics is as vital as new terminals, aprons, and runways.