Predictive Analytics: Unveiling the Future with Data
Predictive analytics analyzes data to predict the likelihood of certain events happening in the future. Through predictive analytics software, businesses across all industries can understand their customers better and make more informed business decisions.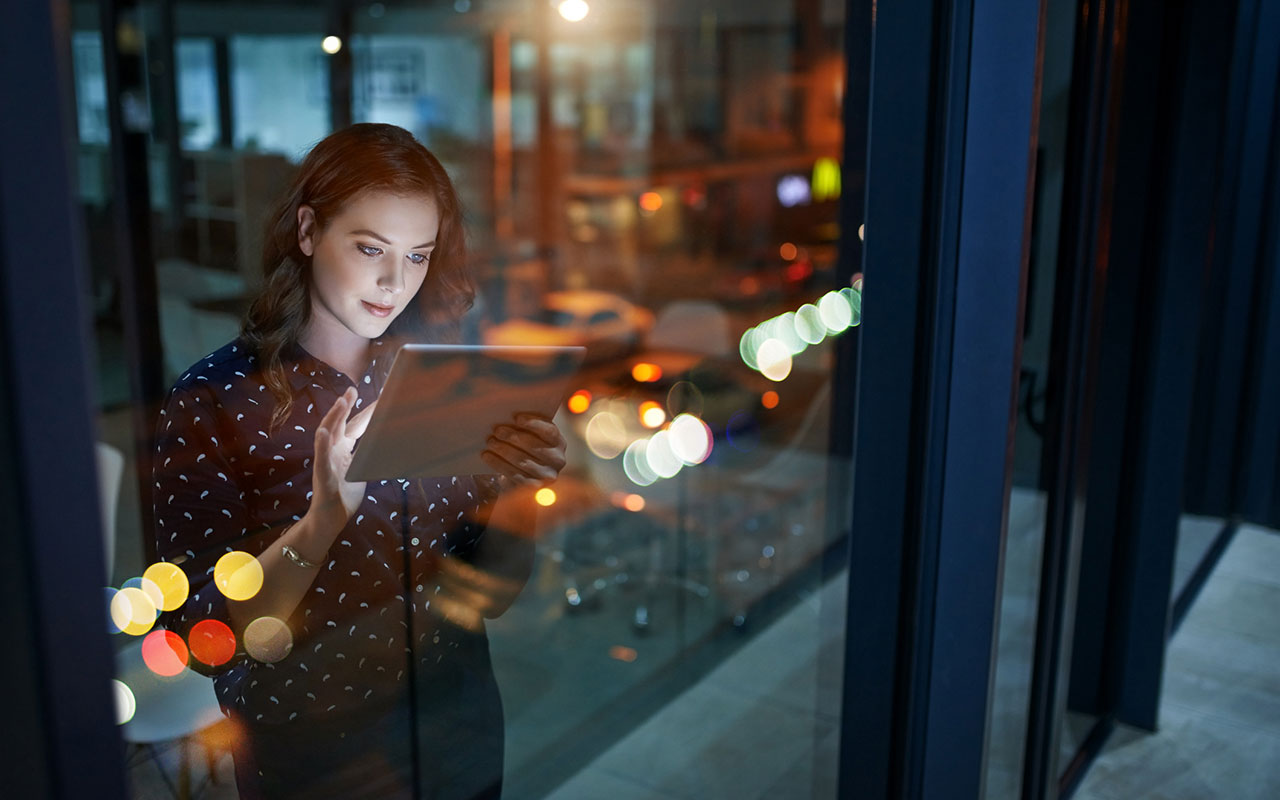
Organizations should take a closer look at predictive analytics to discover the myriad of ways that data and artificial intelligence (AI) can power more personalized customer experiences and enhance brand loyalty and customer retention. From a cost and ROI perspective, the impact and benefits of predictive analytics in customer experience management cannot be ignored.
It’s an opportunity that your company can capitalize on today. According to Forrester, fewer than 10% of enterprises are advanced in their insights-driven capabilities. By equipping your organization with predictive analytics tools, you can gain rich insights into customer behavior, make data-driven decisions, and optimize business operations.
What is Predictive Analytics?
Predictive analytics is a category of data analytics and the process of using data, statistical algorithms, AI, and machine learning techniques to identify the likelihood of future outcomes based on historical data. Put simply: it involves analyzing current and historical data to make predictions about future events or trends.
Advancements in computing power, storage, and algorithms, along with the rise of AI, have made predictive analytics more feasible and accessible to businesses of all sizes. Machine learning algorithms can analyze large datasets quickly and efficiently, enabling businesses to derive insights in real time.
For example, predictive analytics can examine text reviews from customers and predict what steps they are likely to take. Predictive models trained on large datasets of similar text inputs can learn to recognize such patterns and predict future behavior, such as making a purchase or churning.
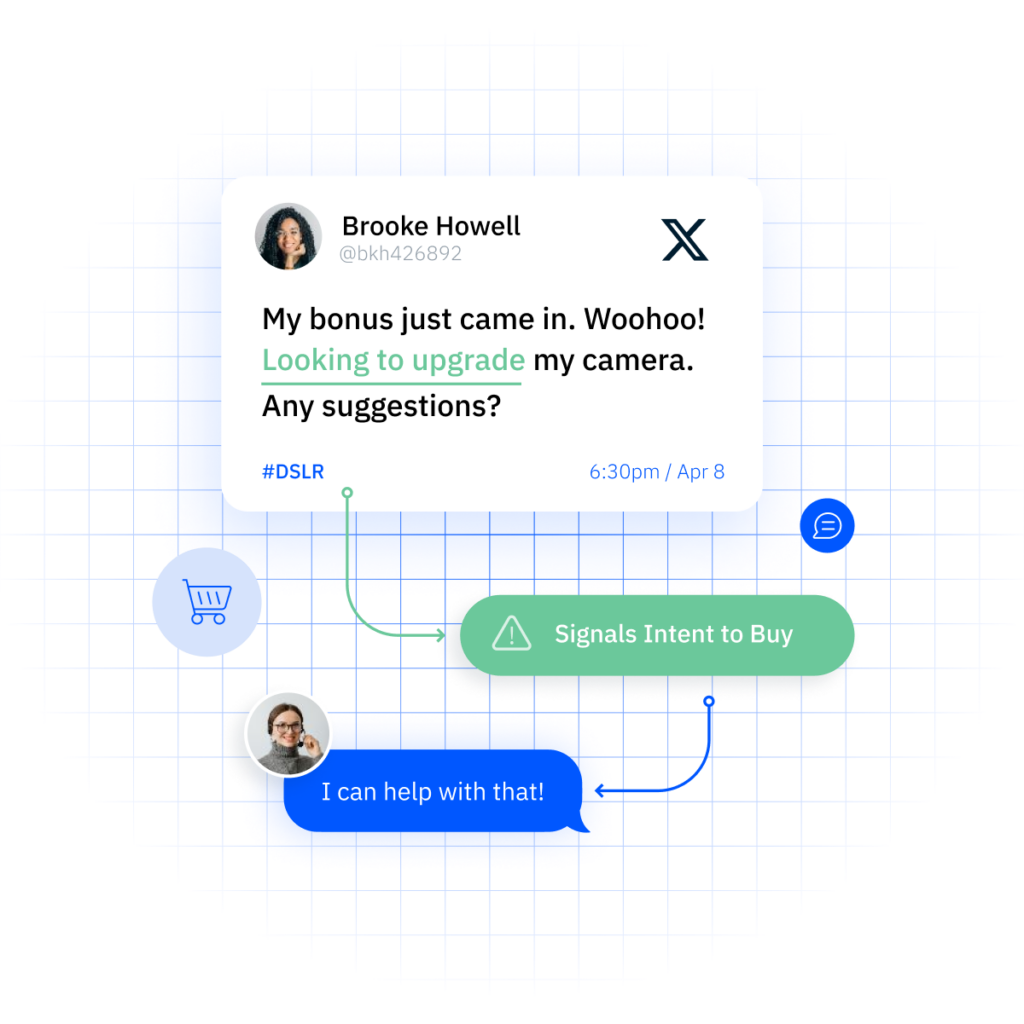
Predictive Analytics vs Prescriptive Analytics
It can be easy to confuse predictive analytics and prescriptive analytics. While they sound similar, they also go hand in hand with each other in practice. These two types of analytics are both designed to provide a comprehensive approach to data-driven decision-making.
As mentioned earlier, predictive analytics is focused on forecasting future events, trends, or behaviors based on historical data. Conversely, prescriptive analytics goes a step further by not only predicting future outcomes but also recommending actions to achieve desired results.
Prescriptive analytics combines predictive models with optimization algorithms and business rules, employing techniques such as simulation, optimization models, and decision analysis. These methods evaluate various possible actions and their outcomes to suggest the best course of action.
Why is Predictive Analytics Important?
Predictive analytics is important because it empowers businesses to make informed decisions that enhance strategic planning and operational efficiency. By analyzing historical data to identify patterns and predict future outcomes, predictive analytics helps organizations anticipate trends, behaviors, and potential risks. This foresight enables businesses to proactively address issues before they become problems, optimize resource allocation, and improve overall performance.
For example, predictive analytics in healthcare enhances patient care by anticipating readmissions and improving diagnostic accuracy. This allows healthcare organizations to proactively manage patient outcomes, allocate resources more efficiently, and implement targeted interventions that reduce hospital stays and associated costs. By identifying at-risk patients early and providing personalized treatment plans, healthcare providers can improve overall patient health and satisfaction, ultimately leading to better clinical outcomes and a more sustainable healthcare system.
Benefits of Predictive Analytics in CX
Predictive analytics is also making an impact on the way companies manage the customer experience. By leveraging data-driven insights from predictive analytics, your company can foster meaningful connections with customers and achieve differentiation in today’s competitive marketplace. The wide-ranging benefits of predictive analytics applications in customer experience management include:
- Enhanced customer loyalty and satisfaction. By predicting what customers want before they even ask for it, your company can provide a proactive and personalized experience that increases satisfaction and fosters loyalty.
- Improve customer lifetime value. Predictive analytics helps identify the most valuable customers and understand their behavior, allowing you to implement strategies that maximize the value these customers bring over their lifetime.
- Reduce customer churn. By identifying patterns that indicate a customer is at risk of leaving, you can take proactive measures to retain them, thereby reducing customer churn.
- Enhance cross-selling and up-selling opportunities. With predictive analytics, marketers can identify which customers are most likely to be interested in additional products or services, creating more opportunities for successful cross-selling and up-selling.
- Accelerate operational improvement. By enhancing the customer experience and making operations more efficient, predictive analytics contributes to accelerated business growth and increased profitability.
What is a Downside of Predictive Analytics?
While predictive analytics can be a powerful tool, organizations need to be aware of the potential downsides and take the proper steps to mitigate or eliminate them. Some of the possible downsides of predictive analytics include:
- Incorrect predictions: Predictive analytics relies heavily on the quality and completeness of the data. Inaccurate, outdated, or incomplete data can lead to wrong predictions, which may result in misguided decisions.
- Ethical and privacy concerns: Using personal data for predictive analytics raises significant ethical and privacy issues. Misuse or mishandling of sensitive information can lead to privacy violations and loss of customer trust.
- False positives and negatives: Predictive models are not perfect and can produce false positives (incorrectly predicting an event will happen) and false negatives (failing to predict an event that does happen). These inaccuracies can lead to inappropriate actions, such as unnecessary interventions or missed opportunities.
These downsides can often be handled and resolved through proper planning, implementation, and maintenance of predictive models. While organizations should be aware of these happenings, they should not deter them from utilizing predictive analytics in their operations.
Examples of Predictive Analytics
Several predictive analytics examples show how the process is being applied by companies looking to better understand their customers, anticipate their needs, and deliver personalized and proactive experiences that drive satisfaction, loyalty, and ultimately, business success.
Predict Behavior and CLV
More and more retail brands are deploying predictive analytics software to forecast customer behavior and monitor market trends.
Retailers can personalize the retail customer experience and increase sales by analyzing information such as past purchase history, browsing behavior, and demographic data. Brands can also leverage predictive analytics algorithms to analyze historical data and market trends, helping predict the optimal price points for products in order to maximize revenue while remaining competitive.
By recommending relevant products, delivering personalized content, and identifying cross-selling and up-selling opportunities based on individual customer profiles and purchase history, brands can create highly personalized retail experiences that drive customer lifetime value (CLV).
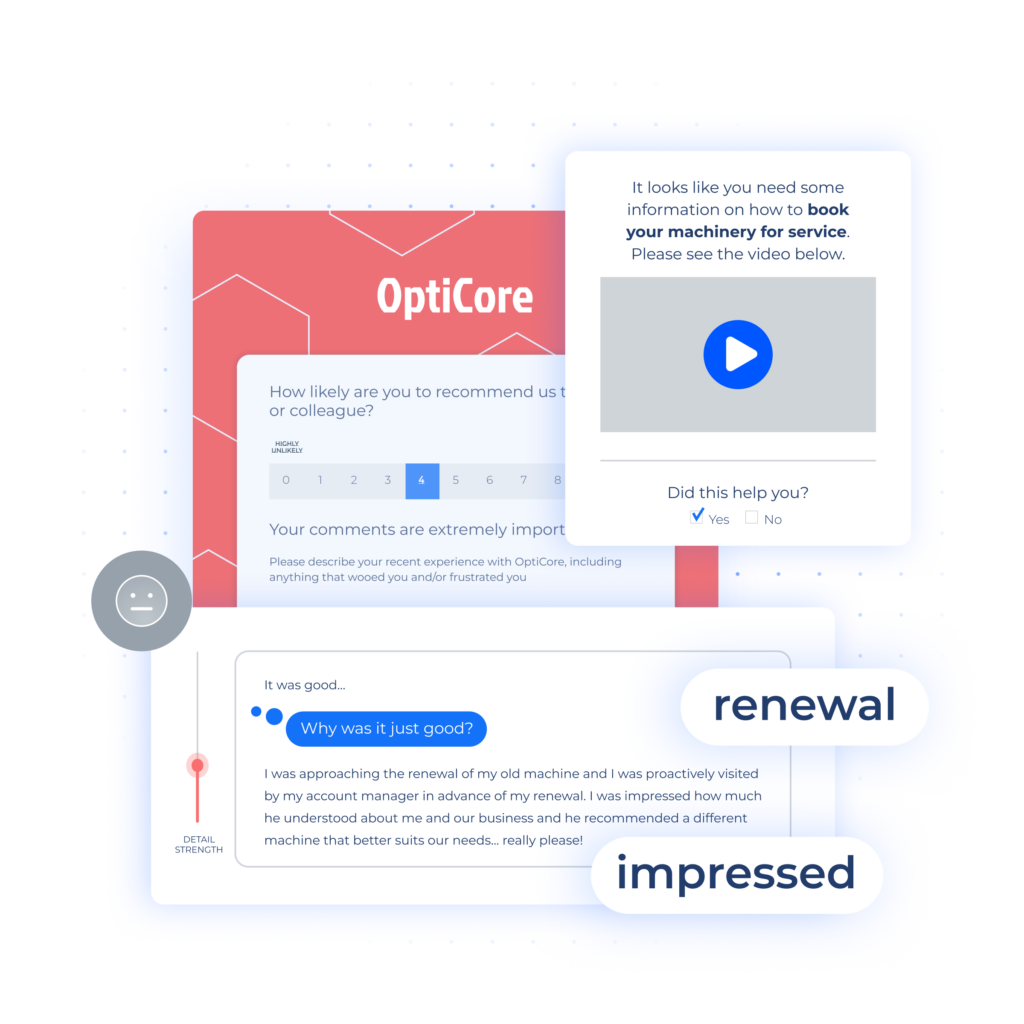
The key is to connect customer experience data from every touchpoint and channel for a complete view of the customer journey. Jim Katzman, Principal of CX Strategy & Enablement for InMoment, suggests that companies should “expand the data sources that you use to understand what your customers are saying and how they perceive you. While surveys will continue to be important, they only give you part of the picture. Expanding your data repertoire to such sources as purchasing data, location-tracking data, web searches, social media, and online reviews is a must.”
The next step is to take a long view when looking at customer relationships. Adds Katzman, “You’ll be surprised at how many brands get caught up in the lure of ‘What can I sell you today?’ without considering what seeds to plant for even more success tomorrow.”
“Equally important is to understand how your competitors view this dynamic and what, if anything, they’re also doing to be proactive when it comes to building lifetime value.
Score Leads by Analyzing Customer Data
Another great application example of predictive is lead-scoring marketers leveraging historical data and machine learning algorithms to predict the likelihood of leads converting into customers. Today more than ever, marketers are empowered to make data-driven decisions when scoring and prioritizing leads, resulting in more effective lead management, higher conversion rates, and improved overall sales and marketing performance.
- Identify Ideal Customer Profiles (ICPs). Predictive customer analytics tools can analyze historical data to identify patterns and characteristics common among high-value customers. By identifying these attributes, marketers can create an ideal customer profile (ICP) that serves as a benchmark for scoring leads based on their similarity to the ICP.
- Assign predictive lead scores. Marketers are also utilizing statistical algorithms to analyze various data points such as demographics, firmographics, online behaviors, engagement with marketing content, and past purchase history to assign a predictive score to each lead. This score indicates the likelihood of a lead becoming a customer based on similarities to past successful conversions.
- Prioritize sales efforts. Marketers can use predictive analytics to prioritize leads based on their likelihood to convert. Leads with higher predictive scores can be routed to sales teams for immediate follow-up, while leads with lower scores can be nurtured through targeted marketing campaigns until they demonstrate stronger buying signals.
- Reduce sales cycle length. Predictive lead scoring enables marketers to identify leads that are further along in the buying process and more likely to make a purchase. By prioritizing these leads for immediate engagement, marketers can accelerate the sales cycle and shorten the time to conversion, leading to faster revenue generation and increased productivity for sales teams.
Harness NLP and Sentiment Analysis to Monitor Brand Reputation
Predictive analytics can also have a significant impact on brand reputation management efforts, helping companies anticipate, monitor, and respond to potential reputation threats more effectively.
Algorithms, for example, can analyze large volumes of data from various sources such as social media and online reviews to gauge customer sentiment toward the brand. By identifying patterns and trends in sentiment data, teams can proactively address emerging issues or negative perceptions before they escalate into major reputation crises.
These analytical techniques help crystallize information contained in reviews into insights — helping companies achieve a more accurate, complete, and unified view of the customer.
With online reputation management software, companies can also analyze customer feedback and sentiment data to identify areas for improvement and proactively address customer concerns. By identifying recurring themes or issues in customer feedback, brands can take corrective actions to improve products, services, and overall customer experience, which in turn enhances brand reputation.
InMoment’s approach is based on machine learning, a method of data analysis that allows companies to find patterns and unlock insights as it is exposed to new review and feedback data. This approach is fast, consistent, and programmable, helping teams quickly understand — at a glance and at scale — exactly what customers are saying. Proprietary relevancy scores for sentiment analysis also provide measurement of positive and negative language, with unparalleled accuracy.
Use AI to Improve Personalization
Predictive analytics empowers companies to better understand their customers, anticipate their needs, and deliver personalized experiences. It’s a particularly powerful tool for curating content based on historical customer data.
One of the best predictive analytics examples comes from streaming giant Netflix, which has a powerful personalized content recommendation engine. The company analyzes user data, including viewing history, ratings, and browsing behavior, to make predictions about what users might want to watch next. This is all reflected as soon as viewers land on Netflix’s home page, which displays content tailored to individual users, improving user engagement and satisfaction.
With predictive analytics, teams can dynamically customize website content, email marketing campaigns, and other communication channels based on individual customer preferences and behaviors. By delivering content that is relevant and timely, businesses can improve personalization, create more engaging customer experiences, and drive higher conversion rates.
Extract Insights from Reviews and Social Media Data
Online reviews and social media data provide a wealth of insights for a business but can be labor-intensive to read through and digest. There are many ways to try to automate this task. Currently, the leading approaches use deep learning models that extract many different kinds of keywords, predict their sentiment, and classify them into relevant categories. This allows companies to improve operations, make better decisions, and elevate the customer experience with data.
Using AI and advanced machine learning techniques, predictive analytics tools can read through thousands of reviews, comments, and other forms of customer feedback in the time it would take a human to read through just a few. The right technology will provide valuable insights, summaries, trends, and statistics that can be applied to support data-driven decision-making and customer-centric innovations.
What to Look for in Predictive Analytics Software
Predictive analytics software allows users to complete predictive analysis. This software can be used by different professionals across many different industries. Predictive analytics software will come with different features, and which specific features will work best for you depends on the goal of your business. However, there are a few foundational features that any successful software will have.
Data Collection and Integration
Data collection and integration is a crucial aspect of predictive analytics software. The feature facilitates the collection of data from various sources, ensuring comprehensive coverage for analysis. It allows users to connect to databases, extract data from APIs, import data from spreadsheets, and integrate data from different systems within the organization.
Data Preprocessing and Cleaning
Another fundamental feature of predictive analytics software is the ability to preprocess and clean data. This allows users to address common data quality issues such as missing values, outliers, duplicate records, and inconsistencies. This feature can also provide automated mechanisms to detect and handle missing values, either by imputing them using statistical techniques or by removing them based on predefined rules. This ensures that the data used for predictive modeling is complete and accurate.
In addition, these features should support outlier detection and treatment. Outliers are data points that deviate significantly from expected patterns. Outlier detection features can identify these outliers and remove them, transform them, or treat them as separate categories based on previously implemented rules or requirements.
Machine Learning Algorithms
Effective predictive analytics software incorporates a wide range of machine learning algorithms, which provides users with powerful tools to build accurate and reliable predictive models. These algorithms form the backbone of a software’s capabilities and enable users to leverage the predictive power of their data.
Model Training and Evaluation
Predictive analytics software should also provide robust functionalities for model training and evaluation, enabling users to build accurate predictive models and assess their performance effectively.
To ensure optimal model performance, software should have options to fine-tune the model’s parameters and settings. Users can experiment with different configurations and optimize the model to achieve the best possible results. This customization capability allows users to adapt the model to their specific use case, maximizing its predictive accuracy and relevance.
Once the model is trained, the software facilitates a thorough evaluation of its performance. Users can assess how well the model generalizes to unseen data by employing various evaluation techniques, such as cross-validation. Cross-validation involves splitting the data into multiple subsets, training the model on a portion of the data, and evaluating its performance on the remaining subset. This process helps estimate the model’s predictive accuracy and identify any potential overfitting or underfitting issues.
Visualization and Reporting Capabilities
Lastly, predictive analytics software should offer robust visualization and reporting capabilities to help users understand and communicate insights effectively, which helps transform complex data into intuitive visual representations and actionable reports.
Users should be able to easily create visual representations of their data, allowing for quick and comprehensive analysis. Visualization options often include bar charts, line charts, scatter plots, heat maps, and geographic maps, among others. These visualizations enable users to identify patterns, trends, and relationships within the data, facilitating deeper insights and understanding.
Furthermore, predictive analytics software should support interactivity in visualizations, allowing users to explore data from different perspectives and drill down into specific subsets of information. Users can interact with the visualizations, apply filters, and dynamically adjust parameters to gain more detailed insights and make data-driven decisions.
Predictive Analytics Implementation and Best Practices
Implementing predictive analytics involves a structured approach to ensure that the data-driven insights generated are accurate, actionable, and aligned with business goals. Here are some key steps and best practices for successful predictive analytics implementation:
1. Define Clear Objectives
Before embarking on a predictive analytics project, it’s essential to clearly define the objectives. Determine what specific outcomes you want to achieve and how predictive analytics will help you reach these goals. Whether it’s improving customer retention, optimizing inventory management, or reducing operational costs, having a clear objective will guide the entire process.
2. Assemble the Right Team
Successful implementation requires a team with diverse skills, including data scientists, data engineers, domain experts, and IT professionals. Data scientists and engineers are crucial for building and maintaining the predictive models, while domain experts ensure that the insights generated are relevant and actionable. IT professionals play a key role in integrating predictive analytics tools with existing systems.
3. Foster a Data-Driven Culture
For predictive analytics to be truly effective, it must be embraced across the organization. Encourage a data-driven culture by promoting the use of data in decision-making processes. Provide training and resources to employees to help them understand and leverage predictive analytics insights.
Jumpstart Your Predictive Analytics Solution With InMoment
The world’s top brands partner with InMoment AI, the leading predictive customer analytics solution, to facilitate the discovery of real-time insights, drive individual customer recovery, and turn unstructured feedback into a predictable source of business growth. To see how what predictive analytics can do for your business, schedule a demo today!
References
Forrester. “Data Governance Unlocks The Impact Of Analytics: Data Strategy & Insights 2023” (https://www.forrester.com/blogs/data-governance-unlocks-the-impact-of-analytics-data-strategy-insights-2023/). Access 03/16/2024.